FedPeWS: Personalized Warmup via Subnetworks for Enhanced Heterogeneous Federated Learning
Nurbek Tastan1 Samuel Horvath1 Martin Takac1 Karthik Nandakumar1,2
2Michigan State University (MSU)
Abstract
Statistical data heterogeneity is a significant barrier to convergence in federated learning (FL). While prior work has advanced heterogeneous FL through better optimization objectives, these methods fall short when there is extreme data heterogeneity among collaborating participants. We hypothesize that convergence under extreme data heterogeneity is primarily hindered due to the aggregation of conflicting updates from the participants in the initial collaboration rounds. To overcome this problem, we propose a warmup phase where each participant learns a personalized mask and updates only a subnetwork of the full model. This personalized warmup allows the participants to focus initially on learning specific subnetworks tailored to the heterogeneity of their data. After the warmup phase, the participants revert to standard federated optimization, where all parameters are communicated. We empirically demonstrate that the proposed personalized warmup via subnetworks (FedPeWS) approach improves accuracy and convergence speed over standard federated optimization methods.
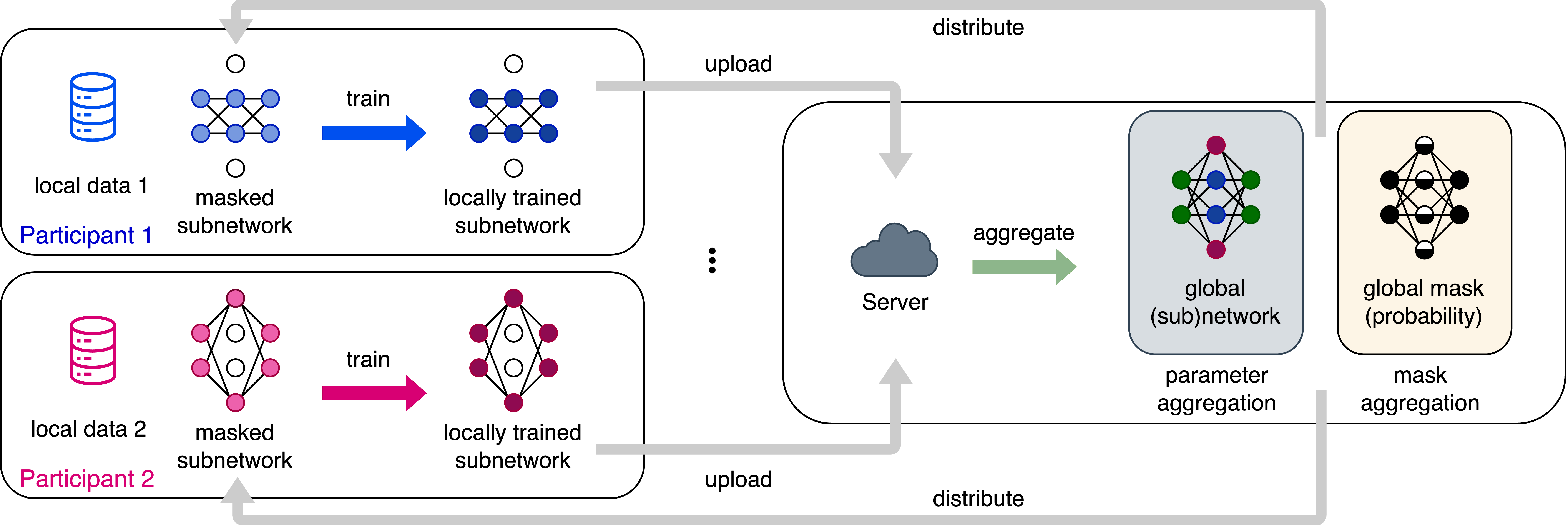
FedPeWS - Main Algorithm
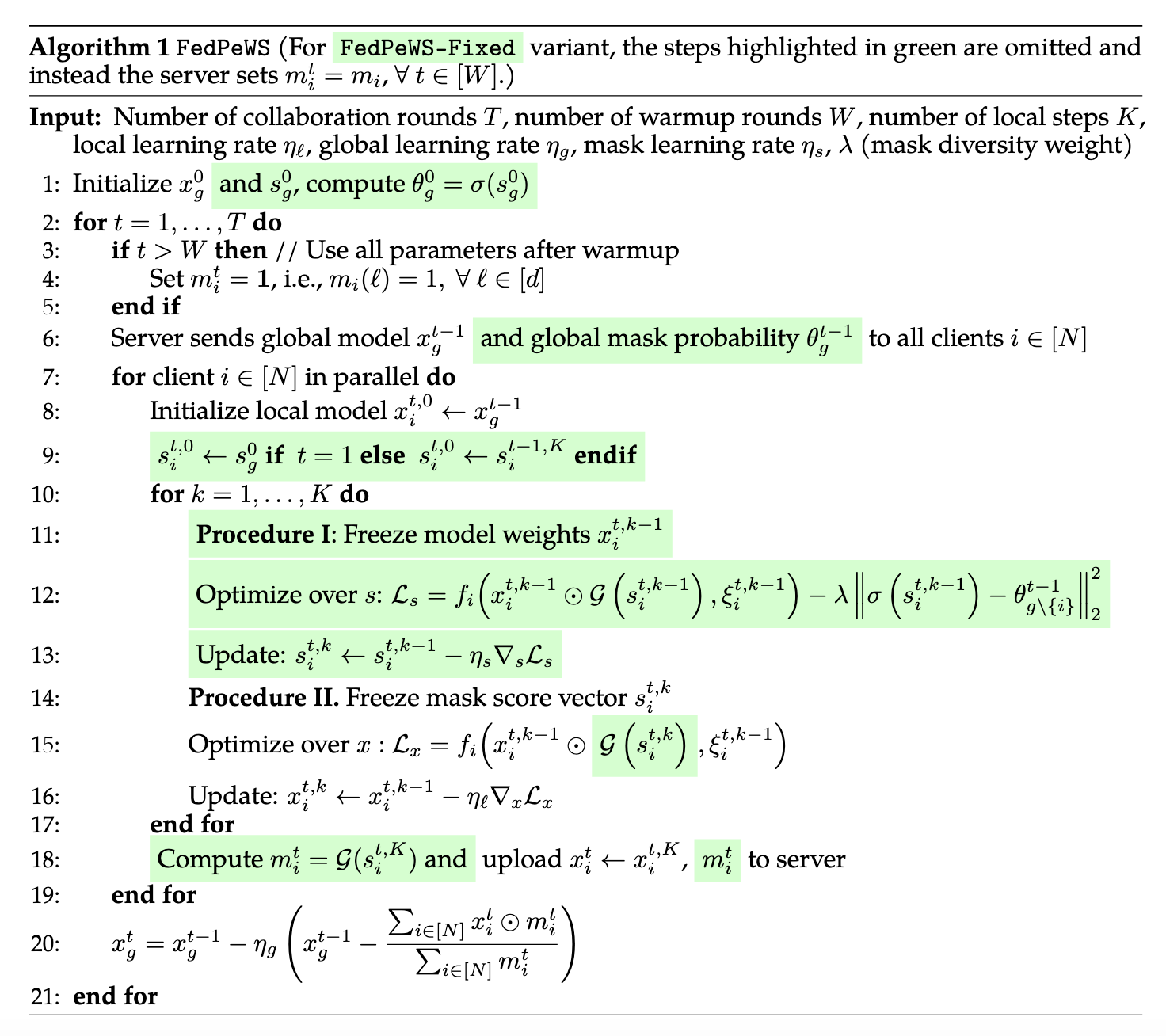
How FedPeWS works?
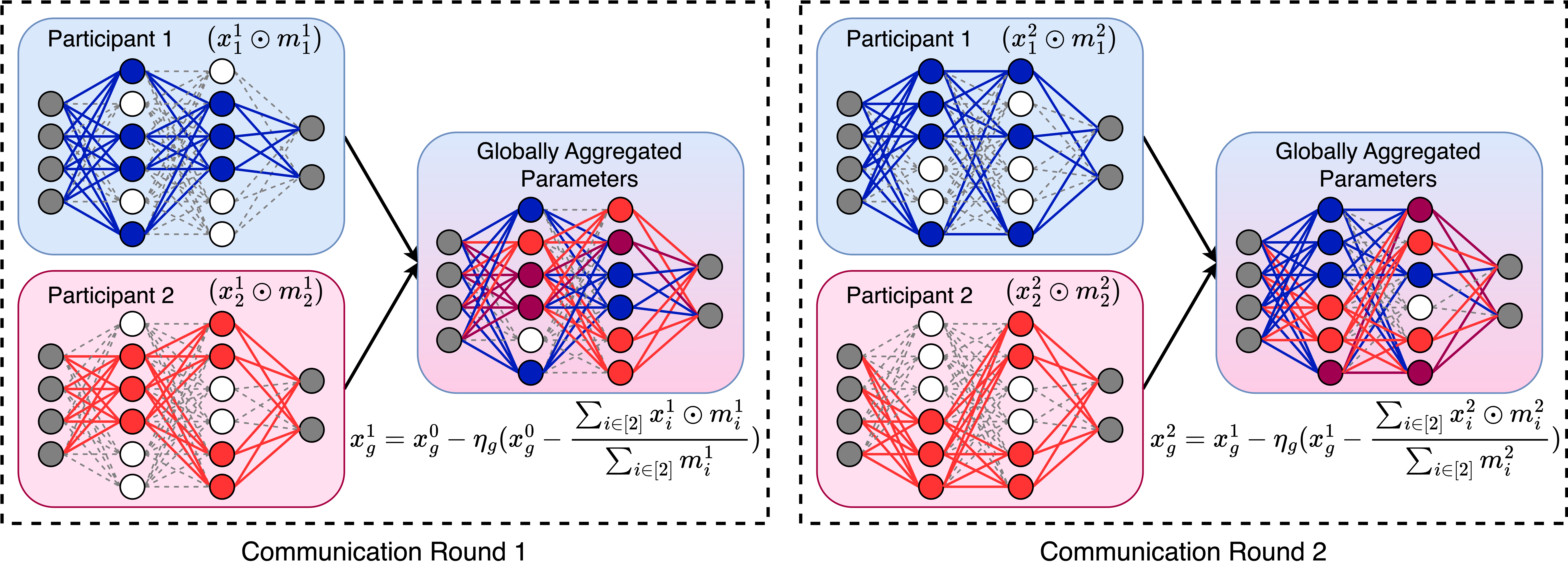
FedPeWS-Fixed. Fixed Mask Generation
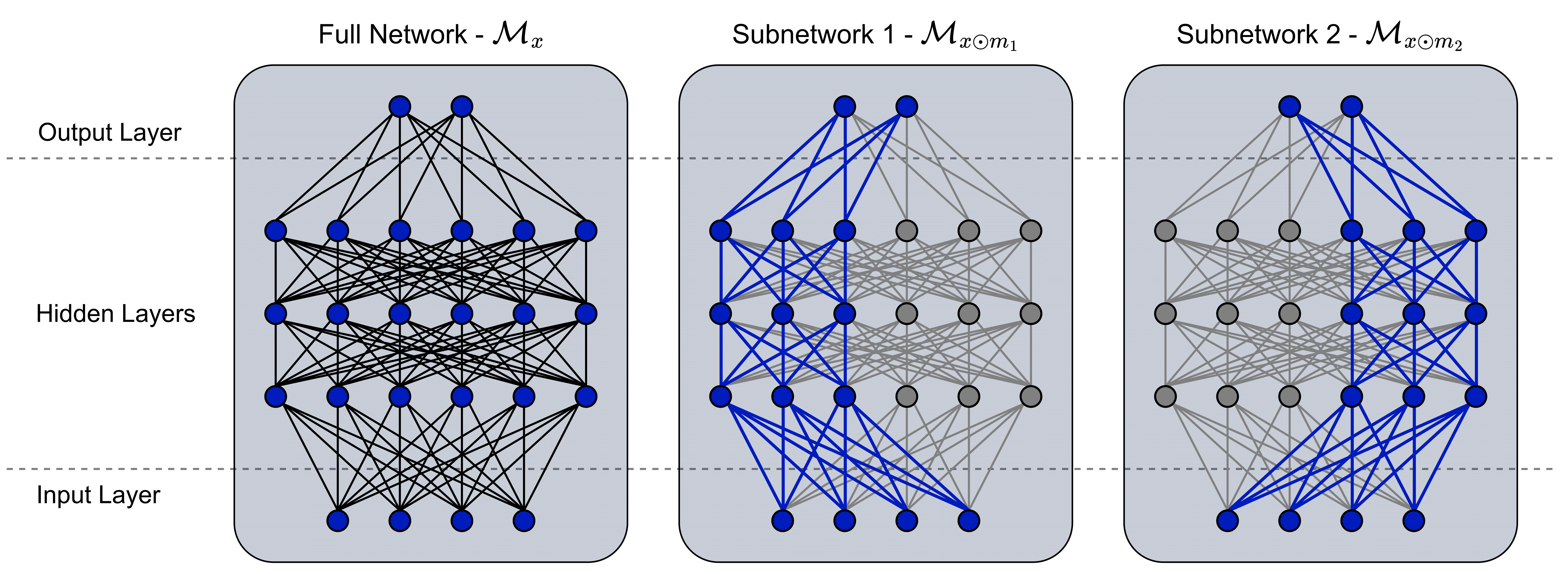
Results: Improved communication efficiency and accuracy
Dataset / Batch size | Synthetic-32K, 32 | Synthetic-3.2K, 8 | |||
---|---|---|---|---|---|
Parameters \( \{ \eta_g / \lambda / \tau \} \) | \( \{1.0 / 5.0 / 0.125\} \) | \( \{0.5/2.0/0.2\} \) | \( \{0.25/1.0/0.1875\} \) | \( \{0.1/2.0/0.1\} \) | |
Target accuracy \( \upsilon (\%) \) | 99 | 90 | 75 | 99 | |
No. of rounds to reach target accuracy | FedAvg | 148 ± 3.79 | 199 ± NA | × | 371 ± NA |
FedAvg+PeWS | 115 ± 7.21 | 182 ± 6.81 | 286 ± 7.93 | 301 ± 10.59 | |
Final accuracy after \( T \) collaboration rounds | FedAvg | 99.94 ± 0.05 | 91.40 ± 7.25 | 67.64 ± 0.90 | 97.33 ± 3.89 |
FedAvg+PeWS | 99.96 ± 0.01 | 99.49 ± 0.60 | 83.50 ± 3.52 | 99.66 ± 0.19 |
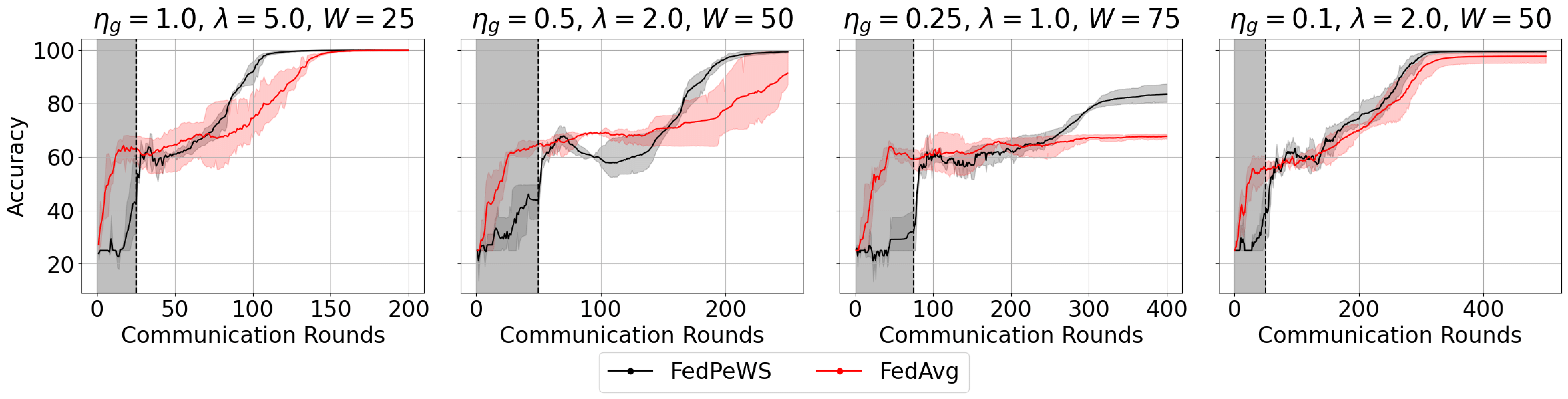
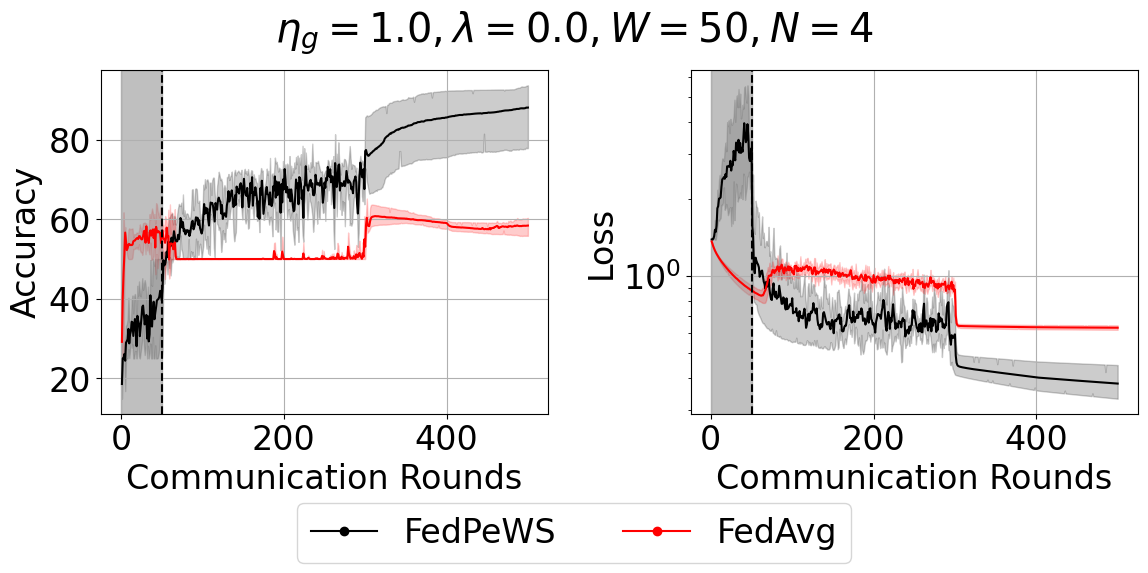
Results: Sensitivity analysis
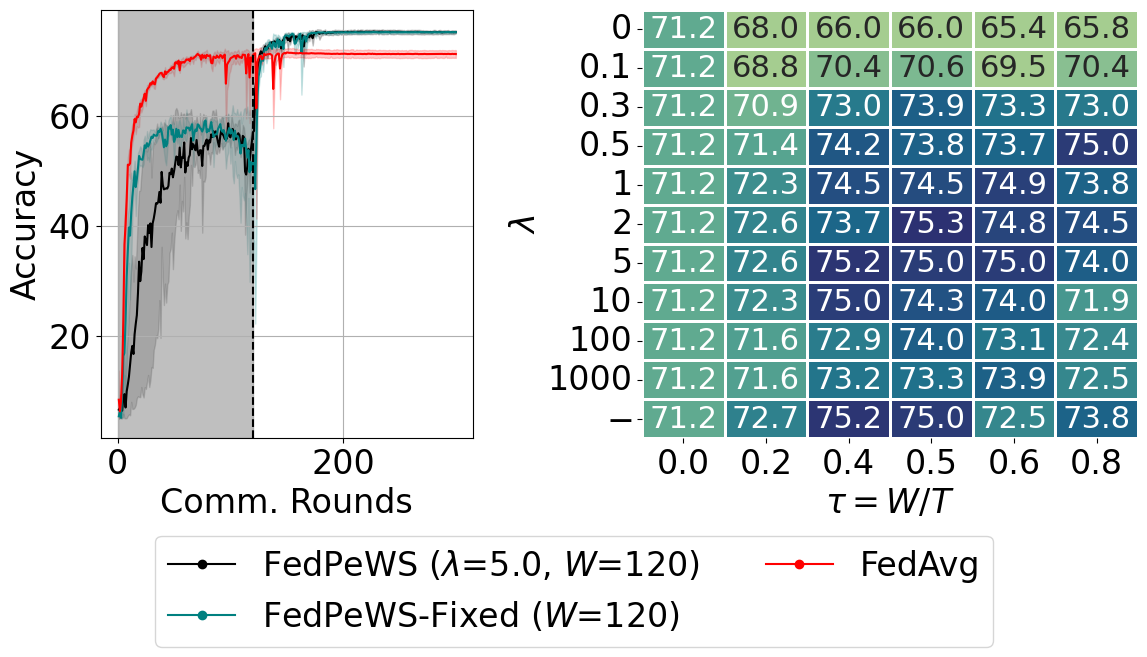
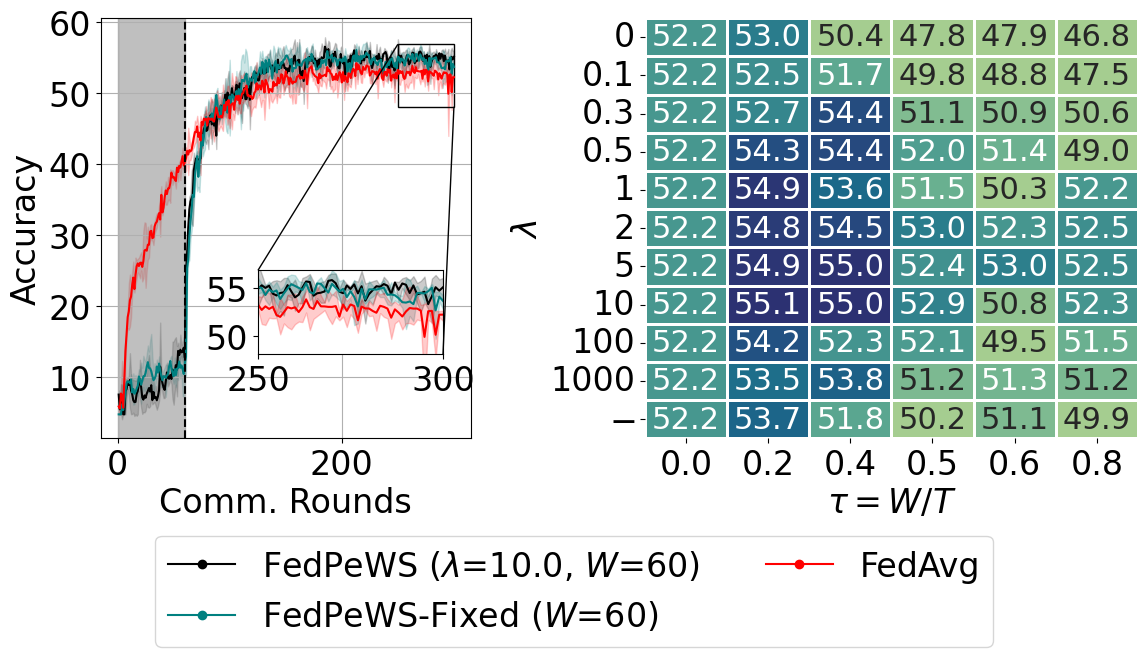
Results: Comparison to SOTA algorithms
Dataset | CIFAR-MNIST | {P-O-T}MNIST |
---|---|---|
FedAvg | 71.78 ± 0.66 | 52.83 ± 1.26 |
FedProx | 72.27 ± 0.88 | 51.28 ± 1.03 |
SCAFFOLD | 71.83 ± 0.24 | 53.05 ± 0.60 |
FedNova | 71.63 ± 0.98 | 53.05 ± 0.83 |
MOON | 71.84 ± 1.09 | 52.10 ± 0.19 |
FedAvg+PeWS | 75.83 ± 0.88 | 55.12 ± 0.56 |
FedProx+PeWS | 75.04 ± 0.85 | 54.67 ± 0.43 |
Conclusion
In this work, we introduced a novel concept called personalized warmup via subnetworks for heterogeneous FL -- a strategy that enhances convergence speed and can seamlessly integrate with existing optimization techniques. Results demonstrate that the proposed FedPeWS approach achieves higher accuracy than the relevant baselines, especially when there is extreme statistical heterogeneity.
Contact
Contact me at nurbek [dot] tastan [at] mbzuai [dot] ac [dot] ae.
Citation
@InProceedings{tastan2025fedpews,
title={Fed{PeWS}: Personalized Warmup via Subnetworks for Enhanced Heterogeneous Federated Learning},
author={Nurbek Tastan and Samuel Horv{\'a}th and Martin Tak{\'a}{\v{c}} and Karthik Nandakumar},
booktitle={The Second Conference on Parsimony and Learning (Proceedings Track)},
year={2025},
url={https://openreview.net/forum?id=iYwiyS1YdQ}
}